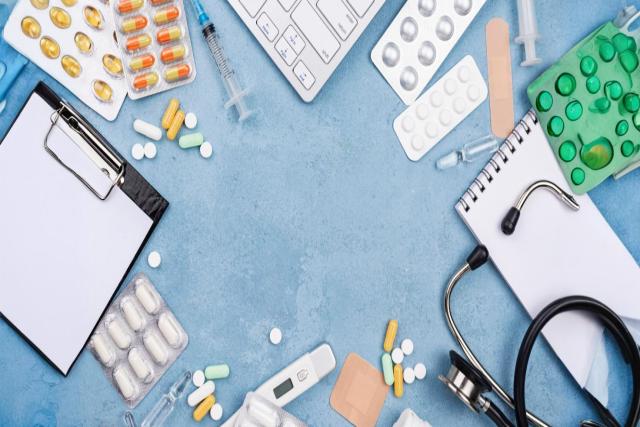
In Pharmacovigilance, AI changes how we monitor and ensure drug security. With the increasing complexity of health data and rapid expansion of pharmaceutical products, effective, accurate, and scalable solutions have never been required. Traditionally, pharmacovigilance (PV) depends on much of manual processes to detect and analyze side effects (ADRS) and other security problems. However, the integration of artificial intelligence into drug safety revolutionizes the region by automating important functions, rapid detection of potential pharmaceutical risks, and improving general patient safety results.
AI in Pharmacovigilance: a new era in surveillance of drugs
AI in Pharmacovigilance has become a foundation stone for modern surveillance of drug safety, which deals with the undesirable event (AE) report and increases data from different sources. Artificial intelligence in drug safety enables automation of many common functions, such as data introduction, unfavorable phenomena that detect and identify potential drug interactions. By treating large amounts of structured and unnecessary data, the AI-Manual systems can quickly identify patterns that will be ignored in another way, ensuring that drug security problems are properly addressed.
Using machine learning algorithms, AI can predict side effects based on historical health data, offering a more proactive approach to pharmacovigilance. For example, machine learning models are trained on large datasets, such as patient health records and medication information, to predict the likelihood of side effects like acute coronary syndrome (ACS) and identify which medicines may contribute to these outcomes. By determining the contribution of specific medications, AI provides a broad, evidence-based method for assessing drug safety, making it an invaluable tool for both pharmaceutical companies and regulatory bodies. These advancements in Pharmacovigilance Industry enhance the ability to detect and mitigate potential risks earlier in the drug lifecycle, ultimately improving patient safety and ensuring compliance with global regulatory standards.
Machine learning in pharmacists: Increase signal detection
Machine learning Pharmacovigilance is one of the most important innovations in the region. Traditional pharmacovies depend on spontaneous reporting systems, where health professionals and patients report side effects. However, with the pure data, manual analysis is often time -consuming and disabled. The machine learning addresses this challenge in automatic pharmacovigilan data processing and analysis.
One of the key advantages of using machine learning in pharmacovigilance (PV) is its ability to detect subtle data patterns and identify emerging safety signals. By analyzing large datasets from electronic health records, clinical trials, and spontaneous reports, AI algorithms can highlight potential risks that may not be immediately apparent. This not only speeds up the detection of adverse events but also improves the accuracy of signal detection, reducing the likelihood of false negatives or positives. Additionally, AI-driven systems can continuously learn from new data, ensuring that the pharmacovigilance process remains adaptive and responsive to changing drug safety landscapes. AI systems help ensure pharmacovigilance compliance with international regulations by automating the detection and reporting of adverse events, meeting the stringent requirements set by regulatory bodies like the FDA, EMA, and WHO. This integration enhances the effectiveness and efficiency of safety monitoring, supporting regulatory bodies and pharmaceutical companies in maintaining high standards of drug safety across multiple jurisdictions.
Automating Drug Safety: AI’s Role in Improving Efficiency
AI in healthcare safety monitoring has proven to be a game-changer in automating drug safety processes. Traditional pharmacovigilance workflows often involve labor-intensive manual tasks, including reviewing individual case safety reports (ICSRs), coding adverse events, and categorizing reports. Automating drug safety with AI tools significantly reduces the time and effort required to process these reports, making the entire system more efficient.
For instance, AI systems can automatically extract relevant information from unstructured data, such as free-text clinical notes, social media posts, or medical literature. By converting these unstructured formats into machine-readable data, AI tools help create a more comprehensive and accurate safety profile for each drug. This not only enhances the ability to detect adverse reactions but also ensures that no crucial data is overlooked. Furthermore, AI can perform tasks such as duplicate report detection, categorizing reports as serious or non-serious, and identifying potential drug interactions, all of which contribute to the automation of drug safety monitoring. By leveraging AI to streamline these tasks, pharmacovigilance systems become more efficient and responsive, enabling regulators and pharmaceutical companies to quickly identify and address safety concerns. The role of pharmacovigilance is crucial in ensuring the ongoing safety and efficacy of drugs for public use, as these AI-driven advancements support continuous monitoring and proactive risk management.
AI-Driven Pharmacovigilance: Enhancing Data Accuracy and Reducing Human Error
AI-driven pharmacovigilance offers a high degree of accuracy by reducing human error and bias. In traditional pharmacovigilance, human reviewers may miss critical signals or inconsistently interpret data, leading to delays in identifying safety concerns. By leveraging AI algorithms, these issues are mitigated, ensuring that safety signals are detected promptly and accurately.
AI tools also provide real-time insights, allowing pharmacovigilance professionals to stay ahead of potential risks. For example, machine learning algorithms can flag emerging ADRs as soon as they occur, providing regulatory authorities with the information needed to take swift action. This is particularly important in fast-paced healthcare environments where time is of the essence and delayed responses can have serious consequences for patient safety.
Moreover, AI tools can enhance the robustness of pharmacovigilance systems by analyzing vast amounts of data from various sources, including electronic health records, clinical trials, and post-market surveillance reports. By aggregating data from multiple sources, AI-driven systems offer a more holistic view of a drug's safety profile, increasing the reliability of safety assessments.
The Potential of AI in Adverse Event Detection
AI for adverse event detection is one of the most promising applications of artificial intelligence in pharmacovigilance. AI algorithms can identify patterns of adverse events across large datasets, even in cases where the reactions may be rare or complex. This is particularly important for detecting long-term or delayed effects of medications that may not be immediately apparent during clinical trials.
Using natural language processing (NLP) and machine learning techniques, AI can analyze unstructured data from sources such as clinical notes, patient feedback, and social media. This ability to detect adverse events from non-traditional data sources significantly broadens the scope of pharmacovigilance, enabling the identification of risks that may otherwise go unnoticed. With AI, pharmacovigilance systems can continuously monitor potential safety signals, offering real-time alerts and recommendations for further investigation.
Challenges and the Future of AI in Pharmacovigilance
Despite the clear benefits of AI in pharmacovigilance, there are challenges to its widespread adoption. One of the primary concerns is the need for regulatory approval and validation. Since AI-driven systems rely on vast amounts of data and complex algorithms, ensuring that these tools meet regulatory standards is crucial. Additionally, while AI can automate many tasks, human expertise is still needed to interpret the results and make final decisions about causality and signal detection.
According to Coherent Market Insights (CMI), the global Pharmacovigilance Industry size is set to reach US$14.3 billion in 2032. Global Pharmacovigilance Industry will likely increase at a CAGR of 8.3% during the forecast period.
Moreover, AI tools must be continuously trained and tested to ensure they remain accurate and effective as new data becomes available. This requires ongoing collaboration between AI developers, healthcare professionals, and regulatory bodies to refine and improve AI-driven pharmacovigilance systems.
Despite these challenges, the potential for AI to revolutionize pharmacovigilance is undeniable. As AI technologies continue to evolve, they will increasingly play a central role in drug safety monitoring, offering more efficient, accurate, and proactive solutions to detect and mitigate risks associated with pharmaceutical products.
Embracing the Future of AI-Driven Pharmacovigilance
AI in healthcare safety monitoring and AI-driven pharmacovigilance are revolutionizing the way we ensure drug safety. By automating routine tasks, identifying adverse events, and improving data accuracy, AI is transforming pharmacovigilance into a faster, more efficient, and more reliable system. While there are challenges to overcome, the potential benefits of AI in pharmacovigilance are vast. As the technology continues to evolve, it holds the promise of making drug safety monitoring more proactive, reducing human error, and ultimately improving patient outcomes worldwide.
Source:
Educational Institute: Harvard University
Scientific Journal: European Journal of Pharmaceutical and Medical Research
Government Agency: National Library of Medicine