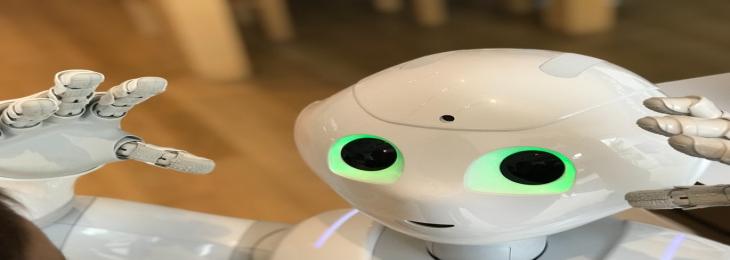
Precise assessment of societal loneliness is discouraging and it is restricted by available tools, which include self-reports. However, according to a new research study initiated by the researchers of University of California San Diego School of Medicine have reported to utilize artificial intelligence (AI) technologies to study natural language patterns (NLP) to detect degrees of loneliness in elderly.
Moreover, it is evident from various sources that there is rising incidence of loneliness that has further augmented rates of suicides and opioid use, increased health care costs, and mortality, in the past decades. Furthermore, according to the experts the scenario has been worse due to COVID-19 pandemic, and it’s associated social distancing and lockdowns.
Ellen Lee, senior author, stated, “Most studies use either a direct question of ' how often do you feel lonely,' which can lead to biased responses due to stigma associated with loneliness or the UCLA Loneliness Scale which does not explicitly use the word 'lonely’. For this project, we used natural language processing or NLP, an unbiased quantitative assessment of expressed emotion and sentiment, in concert with the usual loneliness measurement tools.â€
In the study, researchers considered 80 independent senior residents with an average age of 83 years. Apart from questioning and documenting answers to questions from the UCLA Loneliness Scale, participants were further interviewed by trained study staff with more open-ended conversations, which was evaluated by utilizing NLP-understanding software along with other machine-learning tools.
Researchers observed that lonely individuals expressed sadness significantly to the direct questions about loneliness and had longer responses in qualitative interview. Moreover, women had more acknowledgment towards their loneliness during interviews, whereas men used fearful and joyful words during the interview, in comparison to women. Researchers concluded that the machine-learning models estimated qualitative loneliness with an accuracy by 94%.