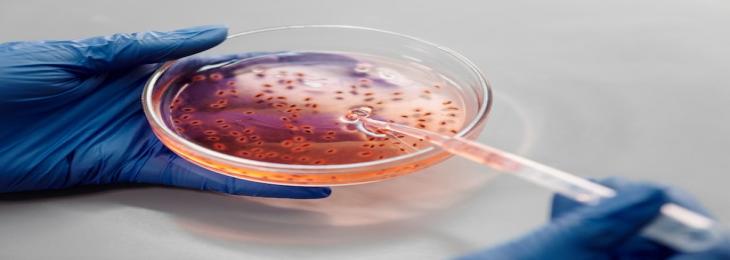
A novel antibiotic that could combat a fatal drug-resistant bacteria common in hospitals and medical offices was discovered thanks to artificial intelligence.
Using an AI algorithm, researchers predicted substances that could combat drug-resistant Acinetobacter baumannii. Abaucin "can effectively suppress" the growth of resistant bacteria on mice's skin, according to a study published this week in Nature Chemical Biology.
Researchers say the technology used to select through tens of thousands of potential therapies can be employed in drug discovery. The paper's lead author, McMaster University assistant professor of biology and biochemistry Jonathan Stokes, said, "There's a lot of fear around AI and I honestly get it. "When I conceive about AI, these models are tools that will better what we do." The Stokes and Broad Institute of MIT and Harvard tested drugs for Acinetobacter baumannii, a superbug that can infect the bloodstream, urinary system, and lungs. This bacteria infects surgical, breathing, and intensive care unit patients.
The CDC recorded 700 deaths from carbapenem-resistant bacteria in 2017 and 8,500 hospital infections. The 7,684 medications and their active ingredients were put to the test against lab-grown bacteria by the researchers. According to Stokes, the lab team employed AI models to find 240 drugs or active ingredients with the highest potential to fight germs. Abaucin, formerly known as RS102895, was discovered to be effective against the superbug after more testing.
According to Stokes, researchers are refining the antibiotic's chemical composition. Abaucin's effectiveness on larger animals and humans will be investigated. According to Stokes, it is essential to remember that a medicine does not merely need to eradicate the bacterium when designing one. In addition, it must be well tolerated by humans, reach the site of infection, and remain there long enough to be effective. Thanks to machine learning techniques, researchers can screen more medications. High-throughput screening can evaluate a few million pharmaceuticals or chemical constituents, whereas machine learning algorithms can evaluate "hundreds of millions to billions" of pharmaceuticals.