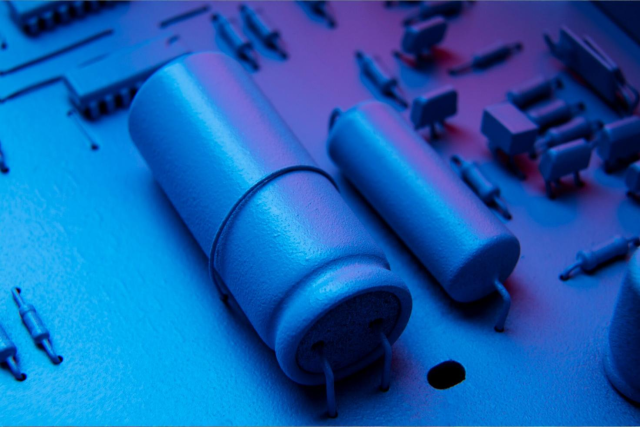
The development of graphical processing units (GPUs) has significantly impacted artificial intelligence (AI) and machine learning (ML), especially in data processing and model training. The global GPU industry was valued at US$37.02 billion in 2022, with an expected CAGR of 12% from 2023 to 2030. The emergence of deep learning (DL) AI, combined with high-performance GPUs, has further enabled real-time image analysis of large datasets and advancements in high-performance computing (HPC) and other AI-driven applications.
Benefits of Using GPUs in AI and Machine Learning
GPUs excel at parallel processing, making them highly effective for AI-driven tasks. Unlike traditional central processing units (CPUs), which perform sequential operations, modern GPUs use thousands of cores to process complex tasks simultaneously.
For instance, deep learning architectures such as convolutional neural networks (CNNs) and transformer models leverage GPU-accelerated AI frameworks for faster training and accurate predictions in natural language processing (NLP) and computer vision AI.
GPU vs. CPU Performance in AI Computing
- Processing Speed: GPUs enable faster AI model training by processing multiple tasks at once, unlike CPUs, which handle tasks sequentially.
- Energy Efficiency: AI-powered GPUs consume less power per computation, making them cost-effective and scalable for AI businesses.
- Memory Bandwidth: High-performance GPUs, such as NVIDIA’s A100 Tensor Core GPUs, offer higher memory bandwidth, ensuring efficient data transfer for AI workloads. For example, NVIDIA’s A100 GPUs provide 20x faster training speeds compared to conventional CPUs, making them ideal for AI research labs and enterprises.
Key Industries Adopting AI-Optimized GPUs
Healthcare: Medical imaging, drug discovery, and predictive analytics (e.g., MRI and CT scans) use AI GPUs for faster diagnosis and treatment advancements.
Finance: Fraud detection systems, algorithmic trading, and risk analysis models rely on GPU-accelerated AI computing for high-speed processing.
Automotive: AI-powered GPUs support autonomous vehicles, enabling real-time object detection, route planning, and safety assessments.
Gaming & Entertainment: GPUs enhance gaming performance by enabling realistic graphics rendering, animation, and real-time physics simulations, creating immersive gaming experiences.
Future Innovations in AI-Optimized GPUs
The future of AI GPUs will focus on enhanced efficiency, advanced neural network processing, and hybrid computing architectures. Some emerging AI GPU trends include:
- Dedicated AI GPUs: Custom GPU architectures designed for deep learning applications, ensuring high-speed computing with minimal latency.
- Edge AI With GPUs: Compact, low-power GPUs optimized for IoT devices and edge computing, reducing dependency on cloud processing.
- Hybrid AI Processors: GPU-CPU hybrid systems that split workloads between parallel processing and sequential computing, improving AI inference performance.
Final Thoughts
The rapid evolution of AI-powered GPUs is redefining the future of machine learning, deep learning, and high-performance computing. As AI models require greater computational power, GPUs will remain essential for scalable AI training and real-time processing. With advancements in dedicated AI GPUs, edge AI, and hybrid architectures, AI-driven industries will continue to thrive, ensuring a smarter, faster, and more efficient digital future.