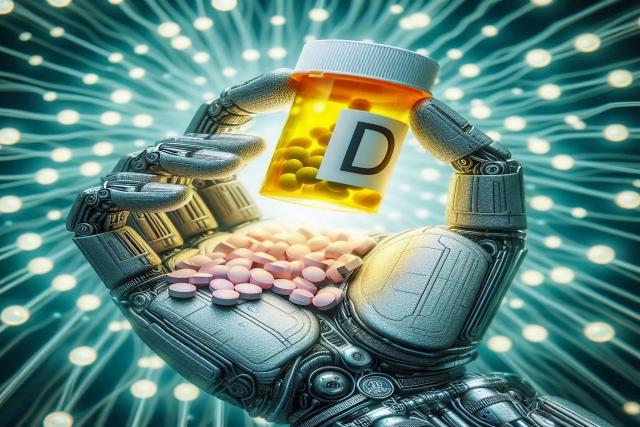
Traditional drug discovery is a complicated, costly, and time-consuming process. In earlier times, this process could take years and billions of dollars. However, despite high investments and years of effort, almost 90% of potential drug candidates used to fail even after they advanced to the phase-I clinical trial. This eventually made room for advanced technologies like artificial intelligence and machine learning.
To overcome challenges of traditional drug discovery methods, subsets of AI like machine learning algorithms and deep learning are being identified as potential solutions. These advanced technologies are transforming the landscape of drug discovery by improving efficiency, reducing costs, and saving time. They also enable breakthroughs in personalized medicine.
Are pharmaceutical giants ready to embrace advanced technologies like AI and ML? Well, the statistics suggest they are. According to Coherent Market Insights, the global artificial intelligence in drug discovery industry is projected to grow at 5.7% CAGR, totaling a valuation of US$ 3,547.4 million by 2030.
Why Machine Learning in Drug Discovery
Machine learning algorithms are being widely used to speed up the drug discovery process. They can analyze large datasets, identify patterns, and make predictions. These ML algorithms are becoming key solutions to enhance efficiency in drug discovery process as well as reduce overall costs and save time.
Machine learning models like deep learning (DL) can process and analyze large amounts of datasets in clinical imaging, virtual screening, bioactivity predictions, and other tasks. Similarly, random forest (RF) models are employed for molecular target identification and feature selection.
Applications of Machine Learning in Drug Discovery and Development
Predictive Modeling
Machine learning algorithms are being used to analyze biological data for identifying potential drug targets like genes or proteins associated with a disease. This, in turn, helps researchers focus their efforts on the most promising avenues.
Machine learning has the potential to predict drug behavior and efficiency through predictive modeling. It can also help in optimizing lead compounds. ML-driven predictive models can forecast compound’s activity, narrowing down the list of candidates for experimentation.
Machine learning algorithms are being widely used in virtual screening. They can evaluate millions of compounds quickly and predict their binding affinity and pharmacological potential.
Compound Synthesis
Machine learning is revolutionizing De Novo Molecular Design by making it faster and more efficient. ML algorithms have the capability to generate novel molecules with desired properties. Generative models like Generative Adversarial Networks (GANs) can understand underlying patterns in molecular structures and generate new molecules.
Drug Repurposing
Machine learning models predict how existing drugs can be used to treat new diseases. This they do by analyzing existing data and identifying potential drug candidates for repositioning.
Toxicity Prediction
ML models can also be employed to predict the toxicity of drug candidates. As a result, they help research to reduce the number of failed clinical trials.
Optimizing Clinical Trials
Machine learning models are used to optimize the design of clinical trials by identifying the most relevant patient populations and predicting the chances of success. By doing so, they can significantly reduce time and cost associated with clinical trials.
Personalized Medicine
Machine learning algorithms are being employed to analyze medical history and genetic information of patients to predict their response to different treatments. In this way, they help researchers to develop personalized treatments that are more effective and have fewer side effects.