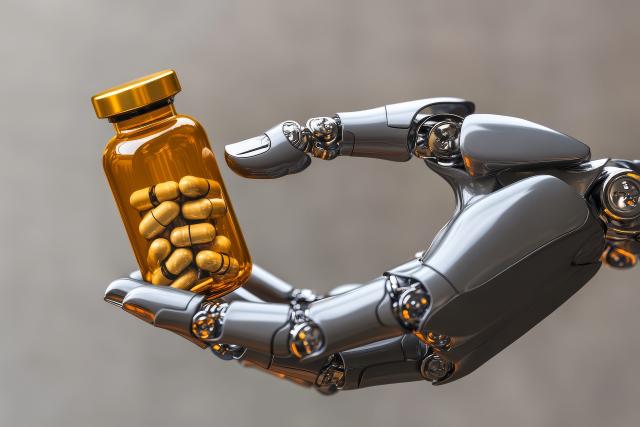
Artificial intelligence and machine learning have thrown a new life into drug development process. These technologies are making strides in the pharamaceutical world by offering advanced techniques for data analysis and decision-making
According to Coherent Market Insights, the global artificial intelligence in drug development industry is set to grow at 5.7% CAGR during the forecast period. totaling US$ 3547.4 million by 2030. Artificial intelligence is automating monotonous operations and providing valuable insights.
Target Identification and Validation
A critical step in drug discovery is identifying and validating potential drug targets. At this stage, AI is being used to analyze extensive biological data, including genomics, transcriptomics, and genomics, to identify molecular targets associated with specific diseases.
AI algorithms can navigate complex datasets quickly and effectively. By doing so, they can significantly improve precision in target identification and reduce the chances of developing ineffective drugs.
Drug Discovery
After identifying targets, the next step is to find a potential drug candidate. Usually, High-throughput screening method is used to identify bioactive compounds. AI complements this process by improving accuracy, reducing false positives, and accelerating the discovery of novel drug candidates with specific properties.
Artificial intelligence can process millions of compounds to forecast their potential to interact with a target. Thus, it helps to facilitate identification of potential drug candidates and reduce the time spent on trial-and-error experimentation.
Lead Optimization
Once the target compound with desired characteristics is identified, drug development enters the lead optimization phase. This is a crucial stage in drug discovery that focuses on refining the structure of lead compound to enhance its potency and other properties.
AI helps researchers in designing molecules that effectively interact with the potential target. It mitigates human bias and data constraints. Generative models like deep learning-based generative adversarial networks (GANs) are being used to design novel drug candidates with desired properties.
Preclinical Testing
Artificial intelligence can process data to predict how a drug will behave in humans. By doing so, it helps researchers to prioritize the most promising candidates for clinical trials.
Clinical Trials
This is an important stage of drug development process. This is because they ensure the safety and efficacy of new drugs before they reach the public. AI has the capability to optimize clinical trial design, patient selection, and data analysis. As a result, it makes clinical trials more efficient and amplifies the chances of success.